Key Takeaways:
- Machine learning and NLP now allow for the analysis of social media to gauge mental health insights.
- These technologies can track daily interactions to monitor and manage stress levels proactively.
- Depending on emotional state changes, interventions might include online resources, counseling, or doctor visits.
Stress is quite common and something many of us face daily. From busy work schedules to personal obligations, we’re under constant pressure. That can take its toll on our physical and mental health.[1]
But what if there’s a way to predict when stress might hit you, and you can deal with it before it becomes a big problem? That’s where machine learning comes in.
Machine learning, a branch of artificial intelligence, is shaking things up in many fields and industries, including healthcare. And within it, it’s especially good in predicting stress levels.[2]
By looking at our daily actions on social media or data from wearables and smartphones, machine learning can connect the dots with known signs of stress and give us valuable insights into our mental health.
This has many applications that could possibly help those in need.
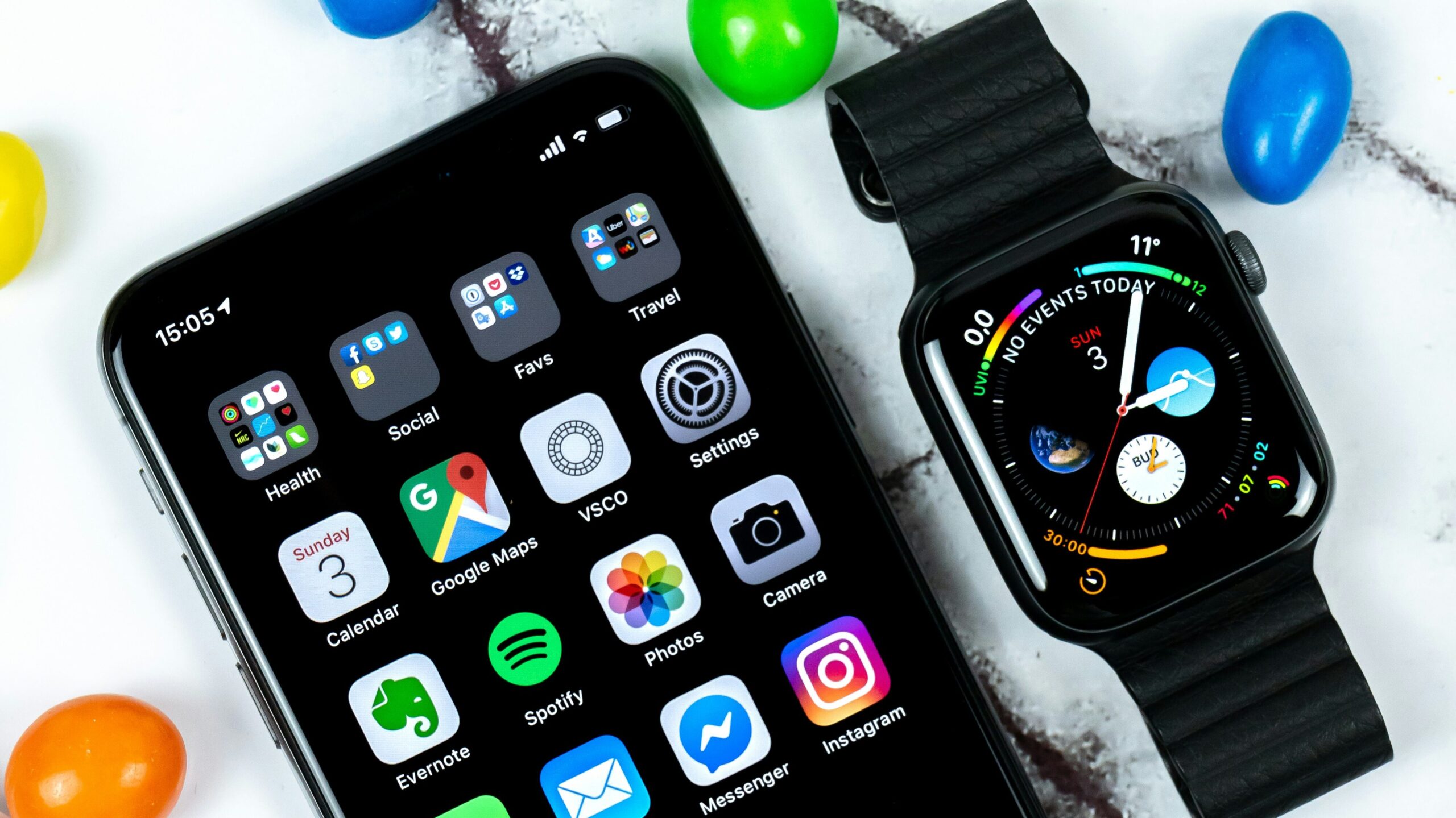
Understanding Mental Health Disorders
Mental health disorders, including anxiety, depression, bipolar disorder, schizophrenia, and ADHD, make significant changes in people’s thinking, emotions, and/or behavior.
They can quickly and easily cause major distress in personal, social, work, and/or family activities.
For some people, even simple duties like getting out of bed or making decisions can feel impossible.
That’s why it’s crucial to get the right diagnosis as fast as possible. And because each person’s experience with these conditions is unique, treatments need to be tailored to fit them like a glove by finding the right combination of therapies, medications, and support.
Using Social Media to Predict Stress Levels
In today’s world, what we do online can show a lot about how we’re feeling. With billions of people on social media, all that data can help us figure out how we’re doing mentally.
By using machine learning (ML) and natural language processing (NLP), it’s now possible to analyze these interactions to predict stress levels and emotional states. This has opened a new frontier in mental health assessment.
Researchers from the Manipal Institute of Technology, India, took this a step further by extending sentiment and emotion analysis to detect individual stress levels based on social media posts and comments.
Utilizing large-scale datasets, particularly from X (Twitter), their study employs machine learning algorithms and the deep learning model BERT (Bidirectional Encoder Representations from Transformers) for sentiment classification.
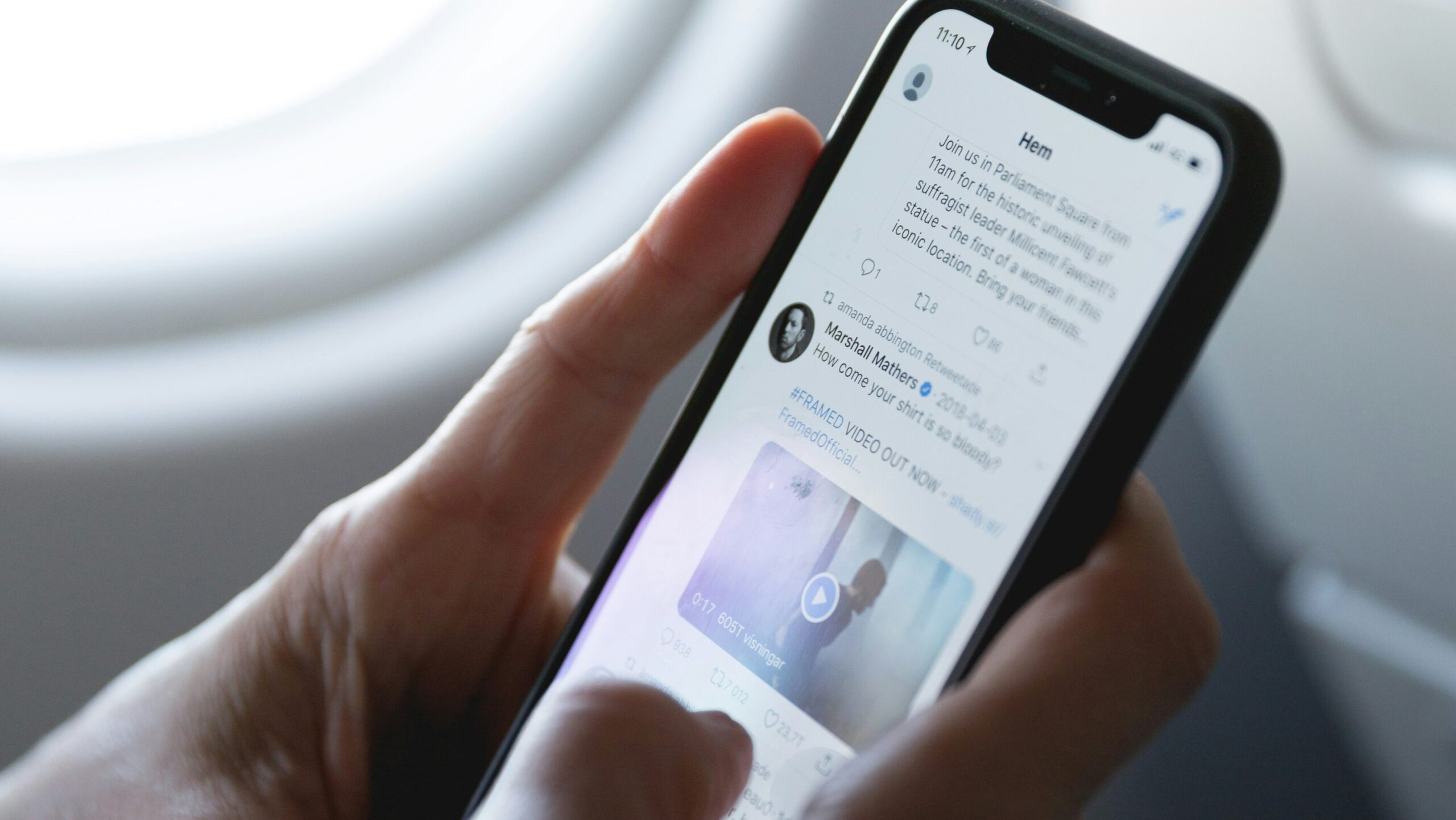
The BERT model, in particular, has shown remarkable proficiency in understanding the context of words in search queries. It’s already surpassing previous models without the need for complex architectural innovations.
And that’s important because researchers can get to gathering and analyzing data right away.
But how can this help regular people in their everyday lives?
Mental health experts can use this powerful tool to assess the mental health and stress of people based on what they’re posting online. In particular, from their comments, posts, likes, etc.
If they spot signs of stress, anxiety, or other issues, they can intervene early and offer support to people who need their help dealing with stress and other emotions.
Here’s how this could work in practice.
Data Collection
One of the first steps in this process is, of course, data collection. The primary source of data is user-generated content on social media platforms, such as posts, comments, likes, reactions, and shares.
Additionally, metadata like timestamps and frequency of posts also provide valuable context.
However, before data collection can begin, researchers need to acquire informed consent from users.
They need to clearly explain to them how their data will be used and ensure that their privacy is protected.
Ultimately, users should have all the control over what data is collected and the option to opt-out at any time if they want to.
Emotion and Sentiment Analysis
Emotion analysis is a way to detect and classify the emotional content of text into categories such as: Happiness, Sadness, Anger, Fear, Surprise and Disgust.
Sentiment analysis, on the other hand, generally categorizes the polarity of the text as: Positive, Negative or Neutral.
Before analysis, text data is preprocessed to improve the quality of the analysis. This includes tasks such as:
- Tokenization (breaking text into individual words or phrases)
- Stemming (reducing words to their root form)
- Removing stop words (common words that add little meaning)
- Handling emojis and abbreviations that are predominant in social media communication
Emotion and sentiment are highly context-dependent. That’s why models must be able to consider the context in which words are used to determine the intended emotion or sentiment accurately.
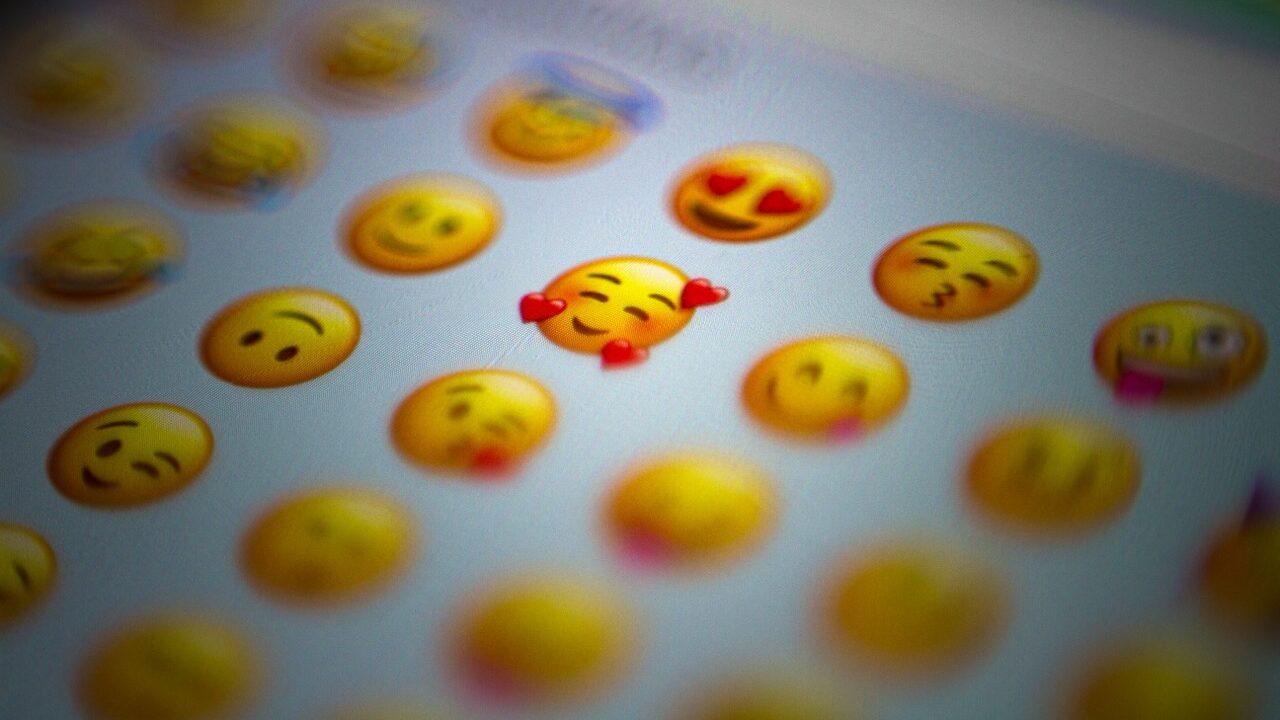
Language is also not always straightforward and is multi-faceted. So, for example, the word “sick” might be negative in a health-related post. It could also be positive when describing someone’s skills or performance.
Stress Level Indicators
Certain emotions and sentiments, especially when people express them frequently or in certain patterns, signal that someone’s dealing with stress or depression.
For instance, if someone posts frequently about how they’re sad or feeling tired all the time, they could be experiencing more than just a temporary bout of sadness. That could be a red flag and a sign of depression, among other things.
There are many other examples:
- If someone loses interest in activities or topics they used to be passionate about, it could be that they’re going through depression
- Changes in their online behavior, like getting into more arguments, might indicate underlying mental health issues
- If their typically positive or neutral posts turn predominantly negative, it could be a sign they’re struggling emotionally
Early Detection
Early detection of stress or depression through social media monitoring relies on identifying when someone starts acting differently from how they usually do online.
Once we know how they normally talk and behave online, we keep an eye on their social media activity.
The system doesn’t rely on a single post but looks for trends. A bad day might lead to negative posts, but a trend over weeks is much more indicative of a deeper issue.
If the system notices a big change that might mean someone’s feeling stressed or depressed, it can send out alerts.
These alerts can go to the person themselves, mental health experts, or someone they trust, depending on how the system’s set up and what the person want.
The key advantage of early detection is that it allows for quick intervention, so people can get help sooner, potentially making a real difference in how bad things get.
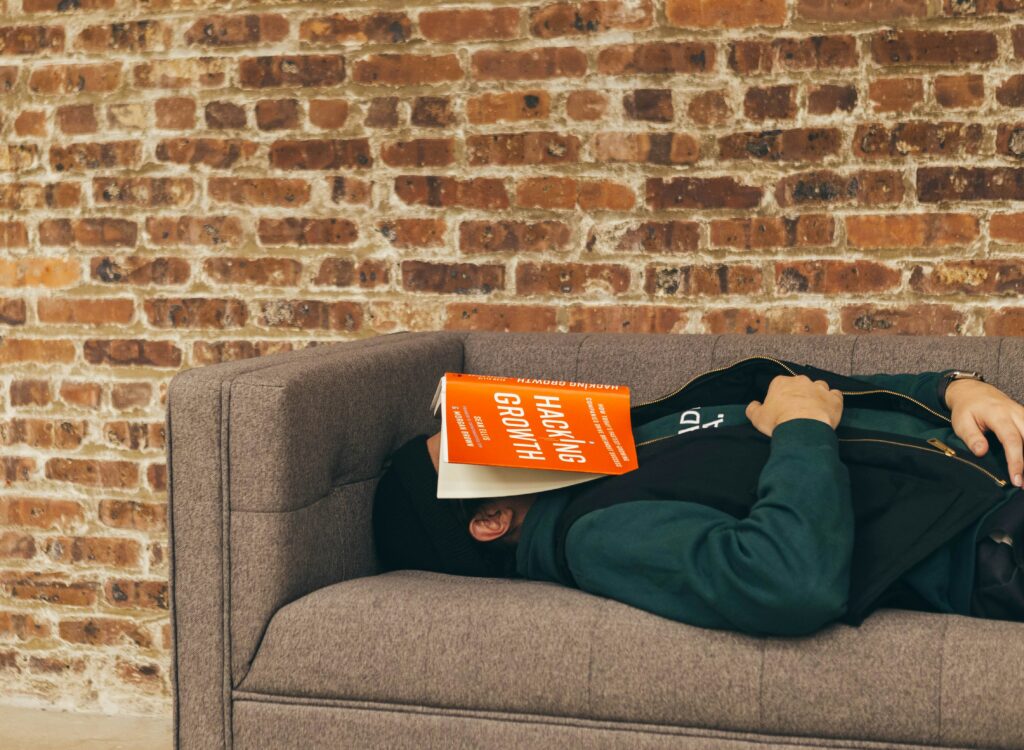
Intervention Strategies
When a potential mental health issue is detected through social media monitoring, intervention strategies can be tailored to the individual’s needs and the severity of the detected changes in emotional state.
This could range from suggesting online resources, offering counseling sessions, or recommending a visit to a healthcare provider.
If there is an immediate risk of harm to the individual or others, emergency services may need to be contacted to provide urgent care and ensure safety.
After the initial intervention, follow-up is key to monitoring the individual’s progress and adjusting the support provided as needed. This might include regular check-ins or additional sessions.
Predicting Stress Levels Through Everyday Devices
Researchers Mohr, Zhang, and Schueller went the other way and started collecting and analyzing data from sensors in everyday devices like smartphones, wearables, and computers to detect human behaviors, thoughts, feelings, and traits.
Wearables, in particular, are very interesting because they are equipped with sensors that can track various types of data, such as physical activity, heart rate, sleep patterns, and more.
In terms of mental health, wearable gadgets gather ongoing, real-time information that shows how someone’s doing both physically and emotionally.
For instance, if a wearable notices changes in how someone sleeps or how active they are, it could signal they’re dealing with depression or anxiety.
The data collected from wearables can be analyzed to identify patterns or markers that are associated with mental health conditions.
This information could then be used to monitor individuals at risk, to understand the progression of mental health issues, or to evaluate the effectiveness of interventions.
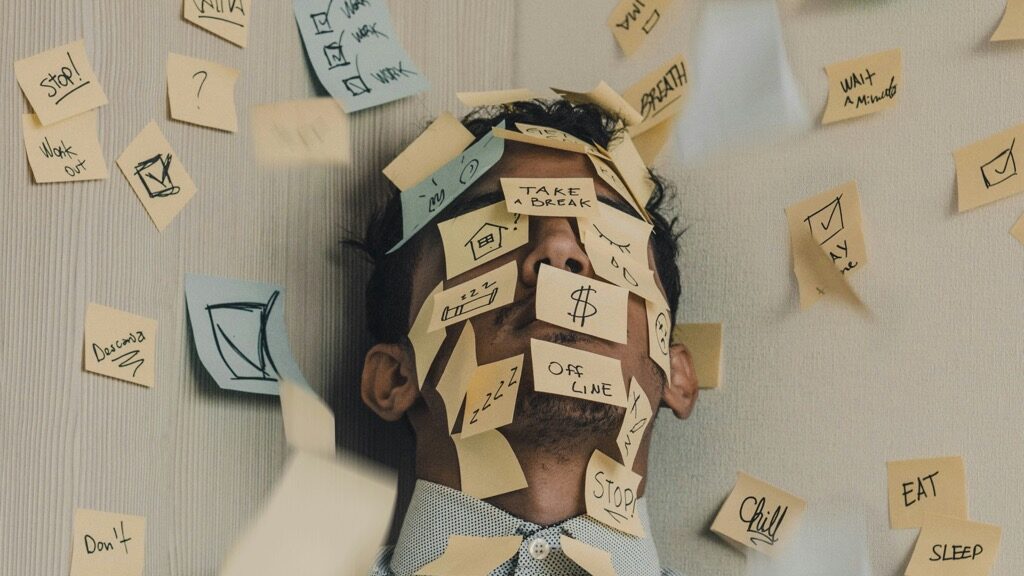
Concerns
Using social media, wearables, or any other tech to keep tabs on mental health brings up big concerns about privacy and ethics. These concerns must be addressed to protect individual’s rights and maintain their trust.
Users need to know exactly what’s happening with their data and agree to it. Data protection measures must be in place to protect sensitive information.
That means preventing unauthorized access, which includes:
- Secure storage of data
- Encryption
- Regular audits to ensure compliance with data protection laws and regulations
There is also a risk that social media posts could be misinterpreted, leading to false positives or negatives in detecting mental health issues.
In other words, mistakes could lead to someone thinking they’ve got a mental health issue when they don’t or missing it when they do.
Professionals need to be careful and seek additional information before jumping to any conclusions.
Sources
1. American Psychological Association. How stress affects your health. 2022. https://www.apa.org/topics/stress/health
2. Alpaydin, Ethem. Machine learning. MIT Press, 2021. Google Books
3. National Academies of Sciences, Engineering, and Medicine; Health and Medicine Division; Board on Health Care Services; Committee on Identifying Disabling Medical Conditions Likely to Improve with Treatment. Selected Health Conditions and Likelihood of Improvement with Treatment. Washington (DC): National Academies Press (US); 2020 Apr 21. 4, Mental Health Disorders. https://www.ncbi.nlm.nih.gov/books/NBK559510/
4. A. Petrosyan. Worldwide digital population. 2024. https://www.statista.com/statistics/617136/digital-population-worldwide/
5. Nijhawan, T., Attigeri, G. & Ananthakrishna, T. Stress detection using natural language processing and machine learning over social interactions. J Big Data 9, 33 2022. https://doi.org/10.1186/s40537-022-00575-6
6. J. Devlin, M. Chang, K. Lee, and K. Toutanova. BERT: Pre-training of Deep Bidirectional Transformers for Language Understanding. Proceedings of the 2019 Conference of the North American Chapter of the Association for Computational Linguistics: Human Language Technologies, Volume 1 (Long and Short Papers), pages 4171–4186, Minneapolis, Minnesota. Association for Computational Linguistics. 2019. https://aclanthology.org/N19-1423
7. Nijhawan, T., Attigeri, G. & Ananthakrishna, T. Stress detection using natural language processing and machine learning over social interactions. J Big Data 9, 33 2022. https://doi.org/10.1186/s40537-022-00575-6
8. Speed, Traci, and J. R DePaulo, Jr.. “Major Depressive Disorder.” Johns Hopkins Psychiatry Guide, 2022. www.hopkinsguides.com/hopkins/view/Johns_Hopkins_Psychiatry_Guide/787049/all/Major_Depressive_Disorder
9. Sarkar, A., Mallik S. S. A Survey of Machine Learning Based Methods for the Diagnosis of Mental Health. Asia-Pacific Journal of Management and Technology (AJMT), 3(2), 39-55. 2022. https://ejournal.lincolnrpl.org/index.php/ajmt/article/view/85
10. Colizzi, Marco et al. “Prevention and early intervention in youth mental health: is it time for a multidisciplinary and trans-diagnostic model for care?.” International journal of mental health systems vol. 14 23. 24 Mar. 2020, doi:10.1186/s13033-020-00356-9
11. Enrique Garcia-Ceja, Michael Riegler, Tine Nordgreen, Petter Jakobsen, Ketil J. Oedegaard, Jim Tørresen. Mental health monitoring with multimodal sensing and machine learning: A survey. Pervasive and Mobile Computing, Volume 51, 2018. https://doi.org/10.1016/j.pmcj.2018.09.003
12. David C. Mohr, Mi Zhang, and Stephen M. Schueller. Personal Sensing: Understanding Mental Health Using Ubiquitous Sensors and Machine Learning. ANNUAL REVIEW OF CLINICAL PSYCHOLOGY Volume 13, 2017. https://doi.org/10.1146/annurev-clinpsy-032816-044949